NFL Draft Analysis
Abstract
This comprehensive study analyzes various aspects of the National Football League (NFL), focusing on the draft process, salary prediction for wide receivers, and factors influencing a team’s playoff chances. Utilizing extensive data from 2009 to 2021, the research employs statistical models, including Random Forest Classification and Logistic Regression, to explore correlations between a player's college statistics, NFL combine performance, and draft position. It also investigates the relationship between wide receiver salaries and performance metrics, and identifies key factors that contribute to a team's likelihood of making the playoffs. The findings offer novel insights into the dynamics of player selection, salary determination, and team success in the NFL.
Introduction
The NFL Draft is a critical process where teams select top college football prospects. Understanding the metrics influencing draft choices and player salaries, as well as the factors determining a team's playoff success, is vital for team management and sports analysts. This study aims to elucidate these relationships using statistical models and data analysis, focusing on quarterbacks, wide receivers, and running backs from 2009 to 2021. The research questions address correlations between players' college performance and draft position, factors affecting wide receivers' salaries, and the determinants of a team's playoff qualification.
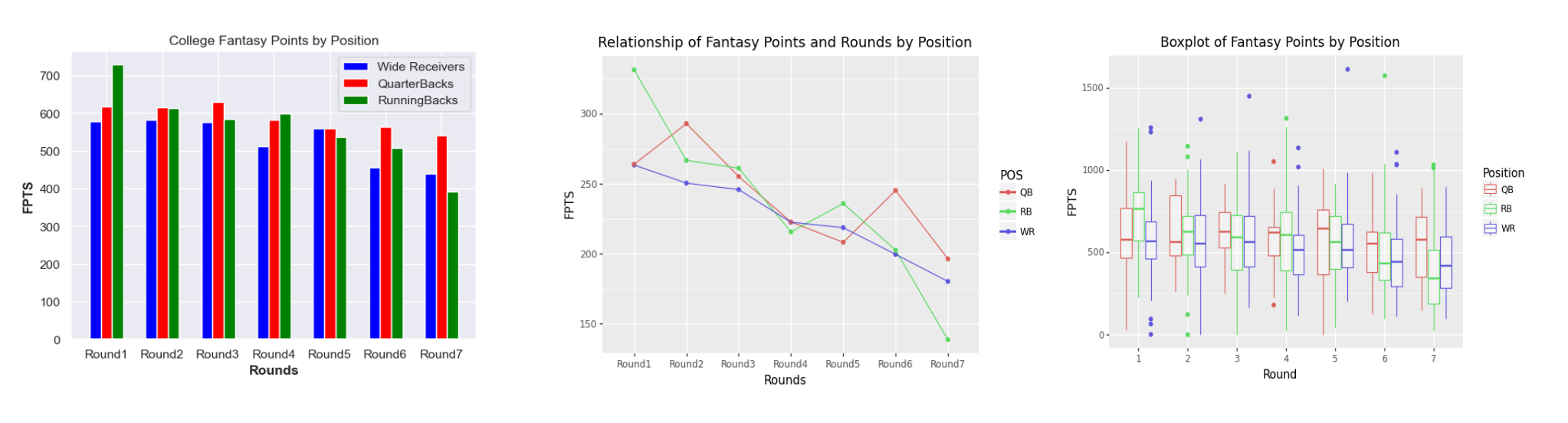
Methodology:
1. Data Collection and Preparation: Data was gathered from various sources, including college football statistics, NFL scouting combine data, and draft positions. The data was cleaned and merged to create comprehensive datasets for analysis.
2. Statistical Analysis: The study utilized Random Forest Classification to identify features impacting draft positions of different player roles and Logistic Regression to predict playoff chances based on team stats.
3. Salary Prediction Model: Data on NFL wide receivers' salaries was scraped and used to build a multiple linear regression model, aiming to predict salaries based on performance metrics.
4. Diagnostic Testing: Various tests, including t-tests, confusion matrix analysis, and the calculation of Variance Inflation Factor (VIF) and Breusch-Pagan test, were conducted to ensure the robustness of the models.
Results
Draft Position Analysis: The models revealed that certain statistics like total yards and touchdowns were crucial in determining a player's draft position. However, the model's moderate accuracy suggests other non-quantifiable factors also play a significant role.
Salary Prediction: The multiple linear regression model showed a strong correlation between wide receivers' performance metrics and their salaries. However, issues like multicollinearity and heteroskedasticity highlighted the complexity of accurately predicting salaries.
Playoff Prediction: The logistic regression model accurately predicted playoff qualifications in about 80% of cases, with key predictors including passing yards per attempt and the number of touchdowns.
Conclusion
The study provides valuable insights into the NFL draft process, player salary determination, and factors influencing playoff success. While certain performance metrics are significant in predicting draft positions and salaries, the complexity and multifaceted nature of these aspects are evident from the moderate accuracy of the models. This underscores the importance of considering a wide range of both quantifiable and intangible factors in player evaluation. Additionally, the findings on playoff predictions emphasize the critical role of strategic plays and quarterback performance in a team's success. This research contributes to a deeper understanding of NFL dynamics and can aid teams in decision-making and strategy formulation.