Heart Disease Factors
Abstract
This study is aimed to explore the interaction effects of various factors that relates to health on the occurrence of heart disease. Additionally, the factors are also explored on the combination of the occurrence of heart disease and stroke on both male and female. The dataset used in this study comprises of 22 categorical variables with various factors involving lifestyle, demographic information, and medical history. This dataset is extracted from Kaggle, and it is called the Heart Disease Health Indicators Dataset. The result of this study provides insights on the relationships of the variables and their impact on heart disease
Introduction
Despite decades of research, heart diseases remain as one of the leading causes of mortality, this accounts a significant portion of the burdens in the field of health care worldwide. Though increased awareness of the factors that account for the risk of heart disease has been developed, the pervasiveness of this disease remains prevalent in our current society. Further research on the contributing factors and the interplay of these factors are required on developing targeted solutions and providing additional counsel for the medical industry. By palliating the morbidity of this disease, we will be able to provide information on the proper lifestyle changes the community should accommodate in efforts of reducing their chances on contracting this disease.
There are various factors that contribute to the increased chances of heart disease. Countless of research has been conducted on the effects of these factors and their risks. Nevertheless, there are less research found focusing on how these factors interact with each other, and how they play a part on the escalation of the probability of contracting heart diseases. By exploring these combined effects, researchers will be able to acquire a better understanding on the complex nature of these factors and how they accommodate to this persistent disease.
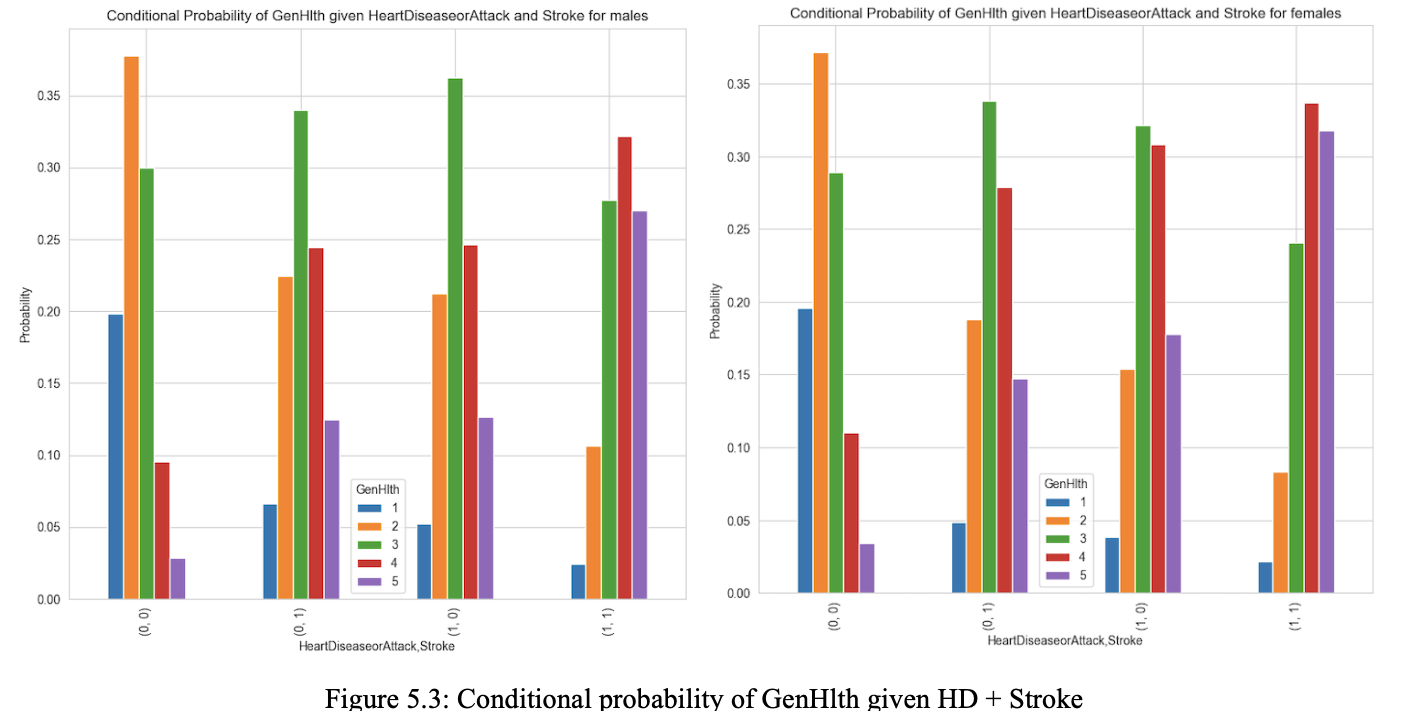
Methodology:
1. Correlation: A correlation plot was created to visualize the possible associations of the variables that could influence heart disease. This was done using heatmaps from the seaborn package. This will help examine the relationships between categorical variables in the dataset.
2. Outliers and Distribution: To identify the potential outliers in this dataset, a series of boxplots was created for each variable, and this allows for the detection of points that are outside of the expected range. Distribution plots was also created to examine the distribution of the variables to find patterns in each variable.
3. Mutual Entropy: Mutual information of heart disease between males and females was conducted for all variables. Additionally, the mutual information of the combination of heart disease and stroke between males and females were also done. This will help provide information about the most significant interactions between the variables.
4. Odds Ratios: The odd ratios for pairwise interactions between variables were also done using a custom created function. These ratios provide additional information to measure the association between the variables and the occurrence of heart disease.
5. Conditional probability: The conditional probability of heart disease on each variable were also done. The results will show the interplay among the factors and their impact on heart disease.
Results
Correlation analysis: Variables like general health, age, difficulty walking, high BP, and stroke showed a strong positive correlation with heart disease. This suggests that the likelihood of heart disease increases when these factors are prevalent.
Outliers and Distribution analysis: Variables such as BMI, mental health, and physical health displayed significant deviations, indicating possible data entry errors or high variability and showed considerable skewness.
Mutual Entropy Analysis: The process revealed distinct mutual information gain patterns between males and females. In the case of males, age and general health were more indicative of a heart disease risk, while for females, general health and difficulty walking were more significant factors. The inclusion of stroke and heart disease as a factor made these interactions slightly more pronounced in females than males.
Odds Ratio Analysis: The odds ratio highlighted significant interaction effects between different variables and heart disease with stroke appearing frequently in these comparisons. The interaction effects were also slightly more pronounced in females than males
Conditional Probability Analysis: It was found that there were noticeable differences in the probability of general health conditions given the presence of heart disease, with variations between genders and between those with and without stroke. The probability of having difficulty walking given heart disease was higher in females, especially when the factor HD is combined with stroke.
Conclusion
This study provides valuable insights into the multifaceted nature of heart disease risk factors, emphasizing the importance of tailored health interventions and the need for further research in this vital area of public health